Data-Driven CX: Using Predictive Analytics for Proactive Customer Engagement
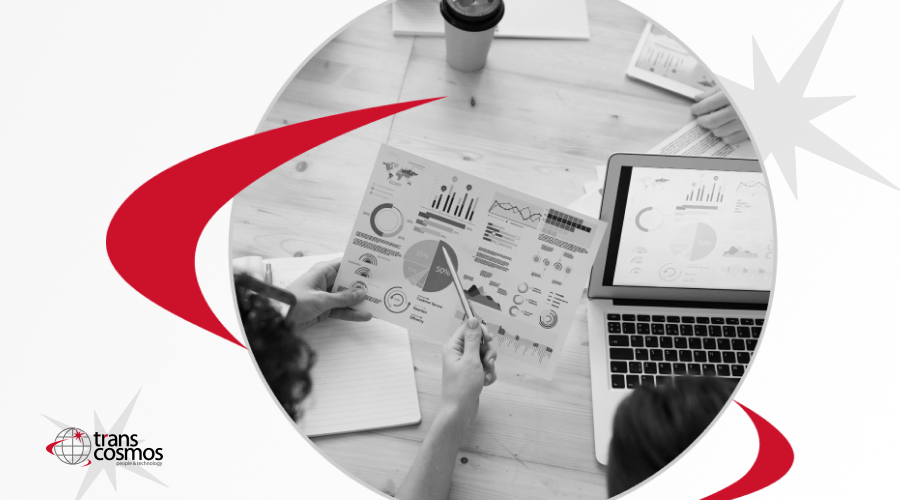
The hyper-competitive market today has become quite demanding. Simply reacting to customer needs is no longer enough. A staggering 86% of buyers are willing to pay more for a great customer experience. This highlights the critical need for proactive engagement—anticipating customer needs and exceeding expectations before issues arise. This is where data-driven customer experience (CX) and predictive analytics come into play, transforming how businesses interact with their customers. This article explores how leveraging predictive analytics empowers proactive customer engagement, leading to significantly improved CX. We will delve into the power of proactive engagement, the mechanics of predictive analytics, practical applications, implementation strategies, and the future of this transformative approach.
Understanding the Power of Proactive Customer Engagement
Proactive customer engagement focuses on anticipating customer needs and providing solutions before problems occur. Unlike reactive engagement, which addresses issues only after they arise, proactive engagement fosters stronger relationships, builds loyalty, and drives increased customer lifetime value. The benefits are substantial: higher customer satisfaction scores, reduced churn rates, and improved brand advocacy.
Consider the difference: A reactive approach might involve responding to a customer complaint about a delayed shipment. A proactive approach would involve proactively notifying the customer of a potential delay and offering a solution, such as a discount or expedited shipping. This demonstrates care and prevents negative experiences before they happen.
The Role of Predictive Analytics in Proactive CX
Predictive analytics uses customer journey maps in combination with historical data, statistical algorithms, and machine learning techniques to identify patterns and predict future outcomes. In the context of CX, this translates to anticipating customer behavior, preferences, and potential issues. Think of it as having a crystal ball for your customer interactions.
Several key techniques power predictive analytics in CX:
Regression analysis: Identifies relationships between variables to predict outcomes (e.g., predicting churn based on customer engagement metrics).
Machine learning: Uses algorithms to learn from data and improve predictions over time (e.g., recommending products based on past purchases and browsing history).
Natural language processing (NLP): Analyzes text data to understand customer sentiment and identify potential problems (e.g., analyzing social media comments to detect negative feedback).
The data sources fueling these analyses are diverse: CRM data, website analytics, social media interactions, customer service records, and transactional data. By integrating these diverse data streams, a comprehensive view of the customer emerges, enabling more accurate predictions.
For instance, imagine a telecommunications company using predictive analytics to identify customers at high risk of churning. By analyzing call logs, usage patterns, and billing information, the system might flag specific customers likely to cancel their service. The company can then proactively reach out to these customers, offering retention incentives or addressing any concerns before they escalate.
Practical Applications of Predictive Analytics for Proactive Engagement
Predictive analytics offers a wealth of opportunities for proactive customer engagement:
Personalized Recommendations: E-commerce giants like Amazon leverage predictive analytics to suggest products customers might be interested in based on their browsing history and past purchases. This personalized approach enhances the shopping experience and boosts sales.
Targeted Marketing Campaigns: By identifying customer segments with high potential for conversion, businesses can tailor marketing messages and offers for maximum impact. This reduces wasted ad spend and improves ROI.
Proactive Customer Support: Predictive analytics can anticipate potential issues before they impact customers. For example, a software company might use predictive analytics to identify users likely to encounter a specific bug and proactively send them a solution or workaround. This prevents frustration and enhances user satisfaction.
Churn Prediction and Prevention: Predicting customer churn is a critical application of predictive analytics. By identifying at-risk customers, businesses can proactively intervene with retention offers, personalized support, or improved service to reduce churn rates. A study by Bain & Company found that increasing customer retention rates by 5% can increase profits by 25% to 95%.
Predictive analytics is revolutionizing how companies manage the customer experience (CX) by leveraging data-driven insights to anticipate customer needs, personalize interactions, and optimize operations. This approach allows businesses to create more meaningful connections with their customers, leading to increased satisfaction, loyalty, and ultimately, business success. Here are specific examples of how companies are using predictive analytics to improve CX:
Predicting Customer Behavior and Lifetime Value (CLV)
Amazon: The e-commerce giant utilizes predictive analytics across various facets of its operations, from its powerful product recommendation engine ("Customers also bought these items") to dynamic pricing adjustments. By analyzing past purchase history, browsing behavior, and demographic data, Amazon personalizes the shopping experience and increases sales.
Retail Brands: Many retail brands are deploying predictive analytics software to forecast customer behavior and monitor market trends. This allows them to identify the optimal price points for products to maximize revenue while remaining competitive.
Scoring Leads and Prioritizing Sales Efforts
Gravity Com Br: This digital marketing agency uses predictive analytics to score leads based on their likelihood of converting into customers. They analyze historical behavior data and assign scores based on factors like company size, industry, and engagement with marketing materials. This helps sales teams focus on the most promising leads, improving customer experience and sales performance.
Harnessing Natural Language Processing (NLP) and Sentiment Analysis for Brand Reputation Management InMoment: This company utilizes machine learning to analyze customer feedback and sentiment data from various sources, including social media and online reviews. By identifying patterns and trends, they help businesses proactively address emerging issues or negative perceptions before they escalate into major reputation crises.
Using AI to Improve Personalization
Netflix: The streaming giant employs a powerful personalized content recommendation engine based on user data, including viewing history, ratings, and browsing behavior. This allows them to predict what users might want to watch next and tailor their home page content accordingly, improving user engagement and satisfaction.
Extracting Insights from Reviews and Social Media Data
Rural King: This farm supply store analyzes massive amounts of unsolicited feedback to unlock the potential of its stores' review data. This allows them to identify areas for improvement, address customer concerns, and create more memorable customer experiences.
Predictive Analytics in Action Across Different Industries
American Express: The financial services company uses predictive analytics to predict potential fraud and identify high-risk customers. This allows them to take proactive action, such as warning customers about potential fraud attempts, and maintain a low fraud loss rate.
DeltaDNA: This gaming analytics platform uses predictive analytics to boost player engagement. Their solution processes data in real-time, enabling games to respond to player behavior and maximize engagement and lifetime value.
Hydrant: The wellness company uses predictive analytics to improve customer retention by identifying customers at risk of churning and developing targeted win-back campaigns. This has resulted in significantly higher conversion rates and revenue per customer.
Automox: The IT automation company uses predictive analytics to expand its outbound marketing and sales efforts. By identifying ideal fit accounts and those in the decision or purchase stage, they have increased sales, closed won deals, and opportunities.
Alleyoop: The beauty brand uses predictive analytics to improve its advertising performance on Facebook. By leveraging first-party data, they create targeted customer audiences and increase incremental sales, Facebook revenue, and return on ad spend.
Donor Bureau: The fundraising company uses predictive analytics to identify loyal supporters and their giving levels, allowing them to personalize campaigns and increase donor interactions and outcomes.
Implementing a Data-Driven CX Strategy
Implementing a successful data-driven CX strategy requires a multi-faceted approach:
Data Collection and Integration: Effective data collection is the cornerstone of predictive analytics. Businesses need to ensure they're capturing relevant data from various sources and integrating it into a unified platform.
Choosing the Right Tools and Technologies: Numerous tools and technologies are available for implementing predictive analytics, ranging from cloud-based platforms to on-premise solutions. The choice depends on the specific needs and resources of the organization.
Building a Data-Driven Culture: Successfully implementing a data-driven CX strategy requires a cultural shift within the organization. Employees at all levels need to understand the importance of data and how it can inform decision-making.
Measuring Success: Regularly monitoring key performance indicators (KPIs) is crucial to assess the effectiveness of the data-driven CX strategy. KPIs might include customer satisfaction scores (CSAT), Net Promoter Score (NPS), customer churn rate, and customer lifetime value (CLTV).
Today's customer experience landscape demands more than just reacting to problems; it requires anticipating needs and building genuine connections. Predictive analytics empowers businesses to do just that, turning data into personalized experiences that foster loyalty, decrease customer churn, and ultimately, drive growth. By embracing data-driven strategies, you're not just improving CX; you're building stronger, more profitable relationships.
Enhance your e-commerce CX with Transcosmos Asia Philippines. We leverage data-driven insights and predictive analytics to drive customer engagement and optimize performance across Australia, New Zealand, and the USA. Contact our sales team to learn more.
Contact Us